Executive Summary
In the dynamic world of AI and machine learning, efficient management of GPU resources in MultiTenant environments is paramount, particularly for Large Language Model (LLM) training. This whitepaper focuses on the pivotal role of ProphetStor’s Federator.ai GPU Booster in transforming GPU resource management for LLM training workloads on large GPU servers equipped with NVIDIA H100s.
Challenges in GPU Resource Management
- Dynamic and Diverse AI/ML Workloads: The varying demands of AI/ML tasks, particularly in LLM training, necessitate an agile and efficient approach to GPU resource allocation, often hampered by static methods leading to underutilization.
- MultiTenant Environment Complexities: The shared nature of GPU resources in Kubernetes cloud environments requires sophisticated management to prevent resource contention and ensure optimal utilization.
Federator.ai GPU Booster: A Game-Changer in GPU Management
- Precision in Predictive Resource Allocation: Federator.ai GPU Booster’s advanced predictive analytics enable exact forecasting of GPU resource needs for various AI/ML jobs, ensuring maximum system efficiency.
- Seamless Kubernetes Integration: Federator.ai GPU Booster’s integration with Kubernetes allows dynamic, automatic GPU resource distribution, essential for high-performing AI/ML workloads.
- Enhanced GPU Utilization with Federator.ai GPU Booster: Federator.ai GPU Booster’s GPU Management and Optimization ensures an increase in GPU resources utilization efficiency, significantly benefiting intensive tasks like LLM training.
- Adaptive Resource Management: In MultiTenant scenarios, Federator.ai GPU Booster’s capability to recommend and adjust GPU resources ensures fair and efficient distribution, maintaining system balance.
- Quantifiable Gains: Implementing Federator.ai GPU Booster’s guidance results in on average 50% reduction in job completion time and more than doubling the average GPU utilization efficiency.
The whitepaper examines how Federator.ai GPU Booster revolutionizes AI/ML resource optimization on GPU servers, particularly for LLM training, marking a significant advance in efficient and powerful AI/ML resource management.
Introduction
In the contemporary sphere of AI and machine learning, managing and optimizing GPU resources is a pivotal challenge, especially in the intricate setups of MultiTenant cloud environments. This whitepaper delves into how ProphetStor’s Federator.ai GPU Booster, equipped with a patented multi-layer correlation technology, is revolutionizing the landscape of resource management for AI/ML workloads, especially on MultiTenant LLM training workloads.
Challenges in GPU Utilization and Management
- Dynamic and Complex AI/ML Workloads: AI/ML tasks, particularly Large Language Model (LLM) training, place heavy demands on GPU resources. Efficiently managing these resources in a dynamic and variable workload environment is a challenge that requires innovative solutions.
- MultiTenant Environment Complexities: Most LLM training workloads run in Kubernetes clusters, where allocating GPU resources across multiple users, projects, and applications is complex. Efficient resource management is critical to prevent conflicts and underutilization.
- Balancing Demand and Efficiency: With fluctuating GPU demands, ensuring a balance between resource availability and efficient utilization is key. Static allocation methods fail to adapt to these changing demands, leading to inefficiencies.
The Federator.ai GPU Booster Advantage
- Predictive Resource Allocation: Leveraging its patented multi-layer correlation and predictive analytics, Federator.ai GPU Booster offers an advanced solution to anticipate and meet the resource needs of various AI/ML jobs. This capability ensures optimal resource distribution, preventing over-provisioning, and enhancing overall efficiency.
- Seamless Integration with Kubernetes: Federator.ai GPU Booster’s integration with Kubernetes allows for dynamic and automatic resource allocation, making it an invaluable tool in managing and optimizing GPU utilization for demanding AI/ML workloads.
- Real-Time Resource Management: In a MultiTenant environment, Federator.ai GPU Booster’s real-time resource adjustment capabilities ensure equitable resource distribution, maintaining system balance and preventing resource contention.
Focus on LLM Training on Large GPU Servers
- Large GPU Infrastructure: Large GPU servers equipped with Nvidia H100 GPUs are tailored for high-demand AI/ML tasks. Their robust architecture makes them ideal for intensive operations like LLM training.
- Enhancing GPU Utilization with Federator.ai GPU Booster: Using Federator.ai GPU Booster for large GPU servers unlocks their full potential. The platform’s predictive and dynamic resource allocation ensures maximum GPU utilization, significantly benefiting LLM training and other AI/ML tasks. From the benchmark results, notably, applying Federator.ai GPU Booster leads to a remarkable 48% decrease in job completion times and an impressive enhancement in GPU utilization efficiency, more than doubling its average performance.
In summary, the integration of Federator.ai GPU Booster with large GPU servers is a game-changer in AI/ML resource optimization. This whitepaper will explore how this integration addresses the pressing needs of efficient GPU resource management and significantly enhances the performance and efficiency of AI/ML workloads, particularly in LLM training.
Federator.ai GPU Booster: Enhancing GPU Management in MultiTenant Environments
In the dynamic realm of AI/ML workloads, particularly in training large language models (LLMs) on advanced GPU servers such as the NVIDIA H100 80GB HBM3 from Supermicro, efficient resource management is crucial. Federator.ai GPU Booster steps into this landscape with a focus on predictive resource allocation and seamless integration with Kubernetes, particularly in MultiTenant settings.
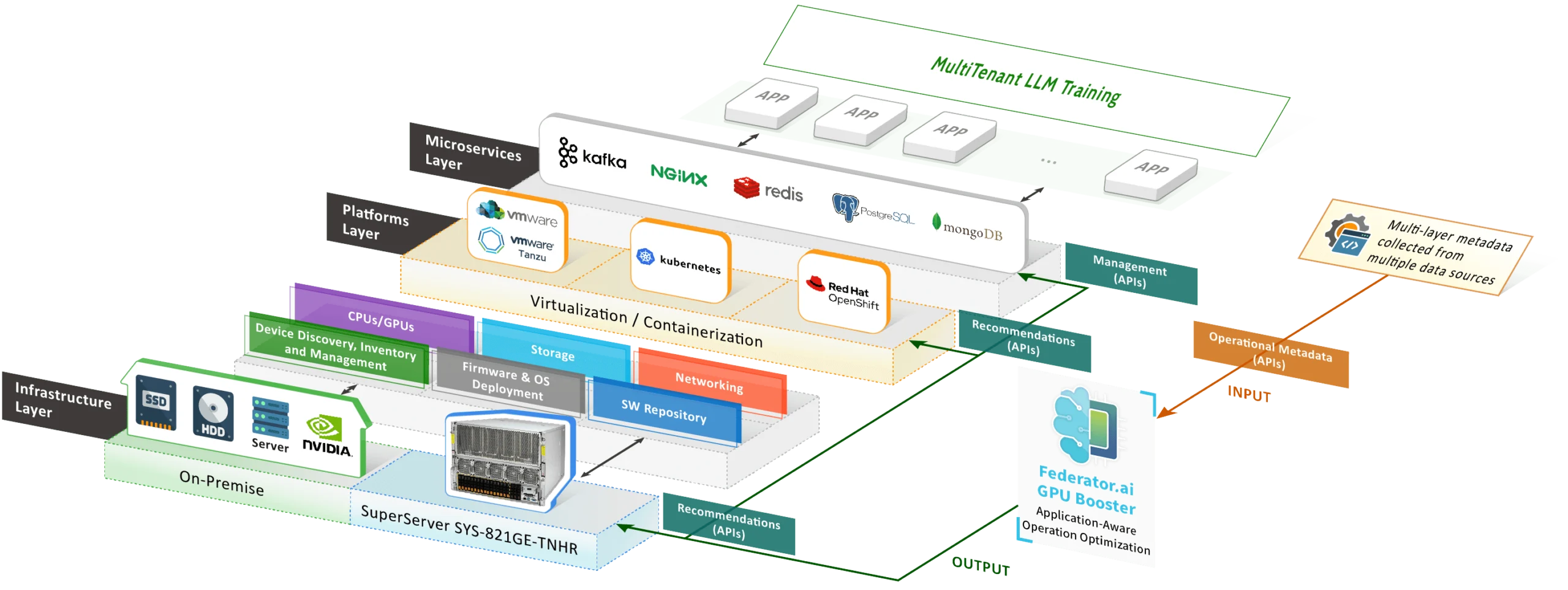
Predictive Resource Allocation
Federator.ai GPU Booster excels in analyzing and predicting the GPU resource needs of various AI/ML jobs, including model training and inferencing, especially for GPT-like models. Its advanced algorithms delve into historical and real-time usage data to anticipate future demands accurately. This foresight allows for several key advantages:
- Customized GPU Resource Recommendations: Based on its analysis, Federator.ai GPU Booster recommends the most suitable GPU resource profiles for each AI/ML job. These recommendations consider the specific computational requirements of the jobs and the available GPU capacities, leading to more effective resource utilization.
- Optimized GPU Resource Configuration: Federator.ai GPU Booster’s insights extend to advising on the optimal configuration of GPU resource profiles. This capability is crucial in environments where multiple AI/ML jobs compete for GPU resources, ensuring that resources are allocated to minimize waiting times and maximize throughput.
Integration with Kubernetes in a MultiTenant Environment
Federator.ai GPU Booster’s integration with Kubernetes is designed to enhance the management of GPU resources in MultiTenant cloud environments. This integration involves several key aspects:
- Dynamic Scheduling and Resource Allocation: Federator.ai GPU Booster interfaces with Kubernetes Scheduler, enabling dynamic scheduling of AI/ML jobs based on predicted GPU resource availability. This approach ensures high GPU utilization and significantly reduces job completion times, even when multiple tenants simultaneously run demanding AI/ML workloads.
- Automated Adjustments and Load Balancing: Federator.ai GPU Booster monitors GPU usage and can trigger real-time adjustments to allocate GPU resources among tenants. This proactive management helps in maintaining an equilibrium, preventing resource hogging by any single tenant, and ensuring fair access to all users.
- Scalability and Flexibility: In a MultiTenant setting, Federator.ai GPU Booster’s scalability is a significant advantage. It can effortlessly manage varying workloads, scaling up or down based on real-time demands and ensuring that each tenant’s requirements are met without compromising overall system performance.
In summary, Federator.ai GPU Booster is a pivotal tool in enhancing the management of GPU resources on large GPU servers equipped with NVIDIA H100 within Kubernetes-driven, LLM Training, MultiTenant environments. Its predictive analytics and dynamic resource allocation strategies ensure that AI/ML workloads are efficiently processed, leading to significant gains in performance and utilization.
Managing Shared Resources
In a MultiTenant environment, Federator.ai GPU Booster tackles several challenges:
- Resource Contention: Multiple tenants vying for the same GPU resources can lead to contention, causing delays or suboptimal performance. Federator.ai GPU Booster monitors resource demands in real time, predicting future needs and mitigating contention by intelligently allocating resources.
- Fair Resource Distribution: Ensuring equitable access to GPU resources for all tenants is crucial. Federator.ai GPU Booster employs sophisticated algorithms that consider each tenant’s workload characteristics and historical usage patterns, ensuring a fair distribution of resources.
- Dynamic Workload Fluctuations: AI/ML workloads with varying computational demands are often dynamic. Federator.ai GPU Booster dynamically adjusts resource allocations in response to these fluctuations, ensuring optimal performance without over-provisioning.
Optimizing GPU Efficiency
Federator.ai GPU Booster enhances GPU utilization in several ways:
- Predictive Analytics for Resource Allocation: Federator.ai GPU Booster predicts the GPU needs of different AI/ML jobs by analyzing historical and current workload data. It then recommends the most appropriate GPU resources, ensuring that each job receives the resources it requires for optimal performance.
- Balanced Workload Distribution: Federator.ai GPU Booster’s integration with Kubernetes allows it to intelligently distribute workloads across the available GPUs. This balanced distribution prevents any single tenant from monopolizing GPU resources, improving overall system efficiency.
- Automated Scaling: Federator.ai GPU Booster can automatically scale GPU resources up or down in response to changing workload demands. This flexibility is key in a MultiTenant environment, where sudden spikes in demand from one tenant can impact the resource availability for others.
- Real-time Monitoring and Adjustment: Federator.ai GPU Booster monitors GPU usage across tenants. It can make real-time adjustments to allocations, ensuring that sudden changes in one tenant’s resource requirements don’t adversely affect others.
In summary, Federator.ai GPU Booster is critical in managing shared GPU resources in a MultiTenant environment, especially when dealing with the complexities of NVIDIA H100 GPUs on large GPU servers. Its predictive analytics and real-time monitoring capabilities allow optimized GPU utilization, ensuring all tenants can run their AI/ML jobs efficiently and effectively.
Use Case Study: AI/ML workload optimization on GPU Server with Nvidia H100 GPUs
This section presents a practical scenario demonstrating how Federator.ai GPU Booster significantly enhances GPU resource management for AI/ML workloads in a Kubernetes environment, mainly focusing on a Supermicro GPU server with 8 Nvidia H100 GPUs.
Scenario Overview
In a Kubernetes cluster with 8 Nvidia H100 GPUs, 20 AI/ML jobs—including model training, inferencing, and GPT-like model training—are scheduled to run concurrently. These jobs vary in GPU resource demands and compete for the available GPU resources.
Case I - Without Federator.ai GPU Booster
Challenges:
- Resource Contention and Underutilization: Due to a lack of predictive resource allocation, multiple AI/ML jobs vie for the same GPU resources, leading to delays in job execution. This contention often results in suboptimal utilization of the powerful H100 GPUs.
- Inefficient GPU Allocation: Each job requests GPU resources without precise knowledge of its actual needs, leading to either over or under-allocation. This inefficiency contributes to longer job completion times and potential GPU resource wastage.
- Job Queuing and Delays: The competition for GPU resources means some jobs cannot start until others are completed, creating a queue and increasing the time required to complete all tasks.
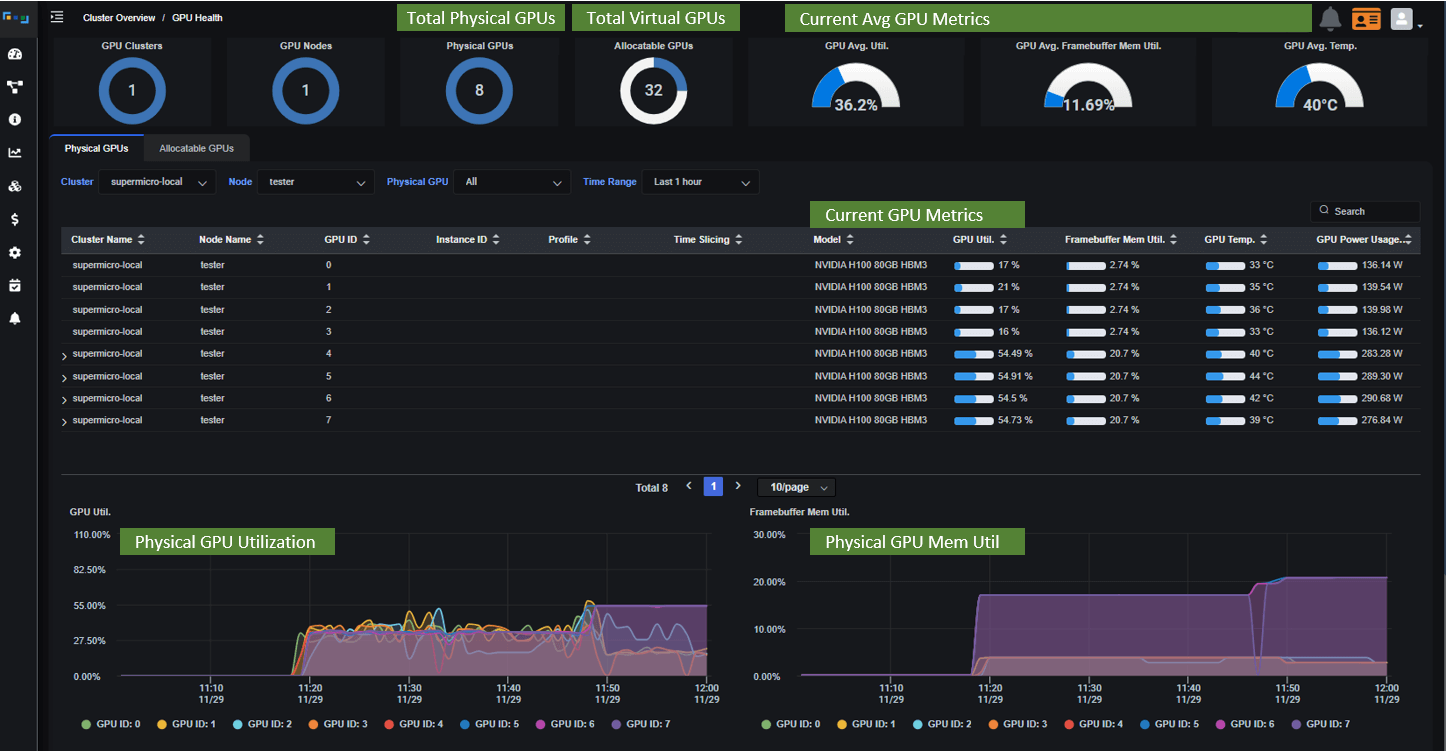
Case II - With Federator.ai GPU Booster Recommendations
Improvements:
- Optimized Resource Allocation: Federator.ai GPU Booster analyzes the GPU resource usage of each AI/ML job. Predictive analytics recommends the most suitable MIG profile for each job, matching their specific resource requirements more accurately.
- Enhanced GPU Utilization: With Federator.ai GPU Booster’s recommendations, the Kubernetes scheduler can allocate GPU resources more effectively. This optimization leads to higher GPU utilization rates, ensuring the powerful Nvidia H100 GPUs are used to their fullest potential.
- Reduced Job Completion Time: Federator.ai GPU Booster’s intelligent resource allocation minimizes job queuing and delays. Assigning the right amount of GPU resources to each job ensures that more jobs can run in parallel, significantly reducing the total completion time for all AI/ML jobs.
- MultiTenant Environment Management: In this scenario, Federator.ai GPU Booster showcases its ability to manage and optimize resources in a MultiTenant setup, ensuring that each tenant or job receives the resources it needs without impacting the performance of others.
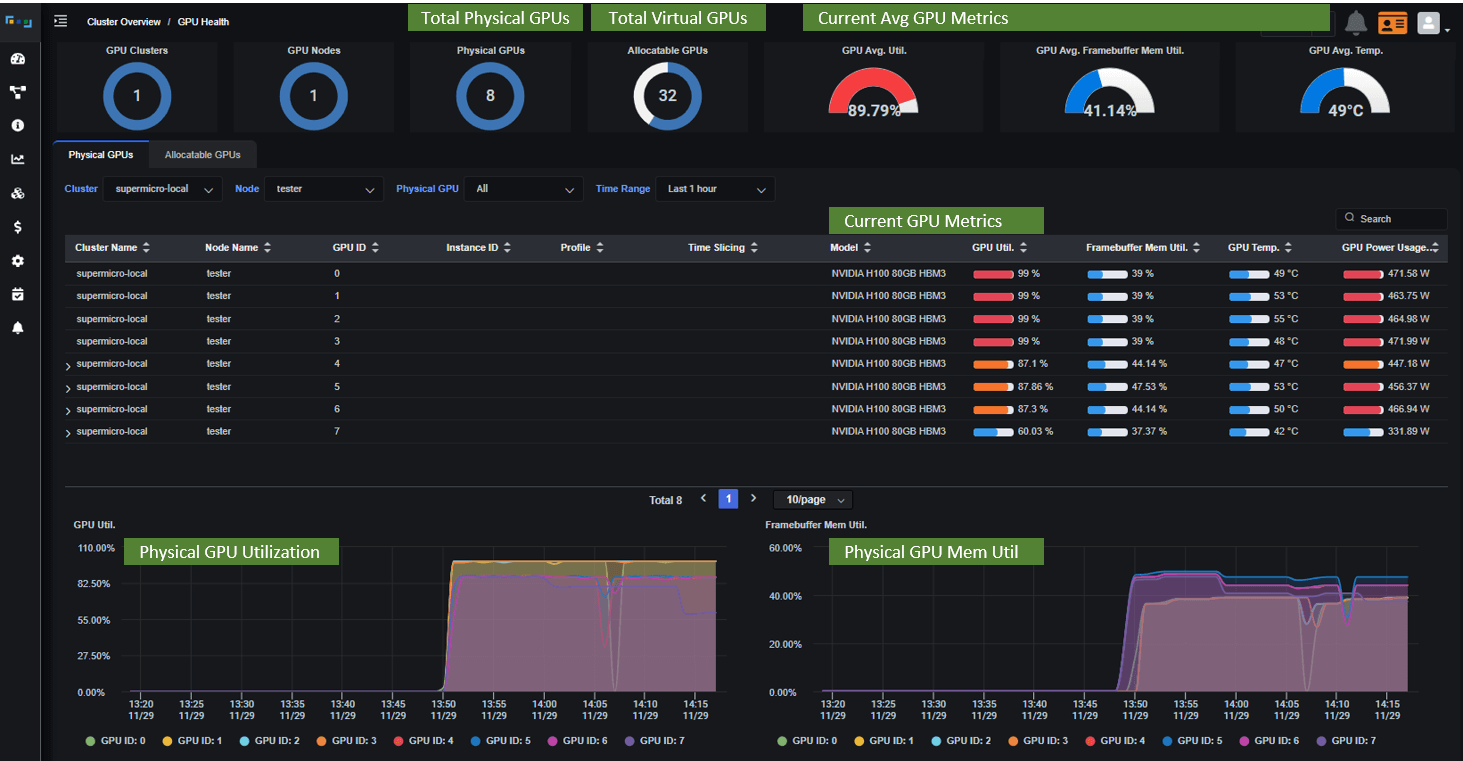
Improvement Analysis
- Total execution time for the 20 AI/ML workloads on a Supermicro server with 8 H100 GPUs before Federator.ai GPU Booster’s recommendations (Case I) is about 114 minutes. Executing the same 20 AI/ML workloads on the same server based on Federator.ai GPU Booster’s recommendations (Case II) is reduced to 59 minutes. This is a 48% improvement in execution time.
- The average GPU utilization on a Supermicro server equipped with 8 H100 GPUs, without Federator.ai GPU Booster recommendations, for the 20 AI/ML workloads (Case I) is 36%. With Federator.ai GPU Booster recommendations applied to the same server for the identical set of 20 AI/ML workloads (Case II), the average GPU utilization increases to 90%. The adoption of Federator.ai GPU Booster has been instrumental in achieving a significant 48% reduction in the time taken to complete jobs, while simultaneously boosting GPU utilization efficiency beyond double its standard rate.
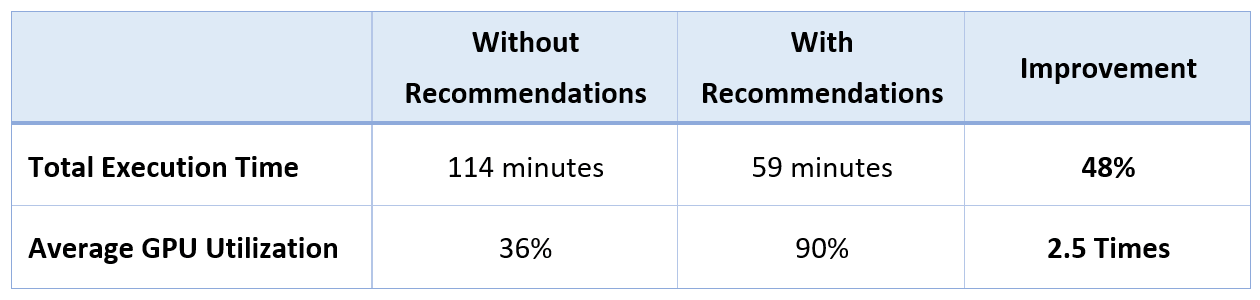
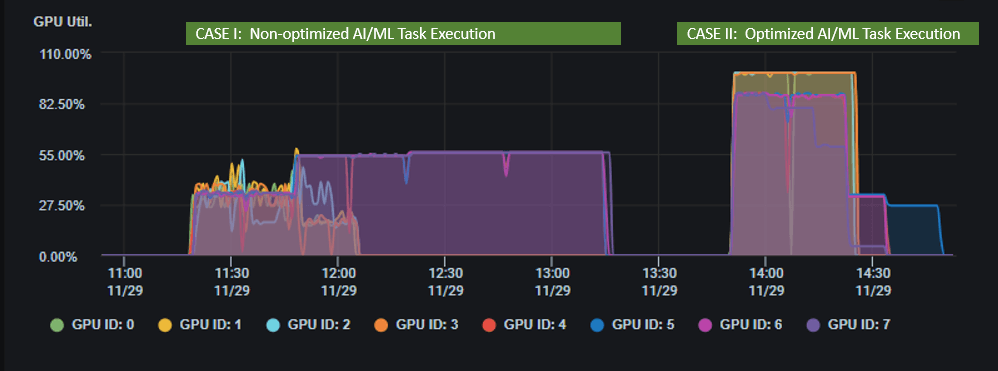
In conclusion, Federator.ai GPU Booster transforms the GPU resource management landscape, especially in complex Kubernetes environments with high-performance GPUs like the Nvidia H100. Its predictive and dynamic resource allocation approach leads to more efficient GPU utilization, faster job completion times, and overall enhanced performance for AI/ML workloads.
Summary: Key Benefits of Federator.ai GPU Booster for large GPU server Optimization
Enhanced Resource Efficiency
- Efficient GPU Allocation: Federator.ai GPU Booster’s predictive analysis ensures optimal allocation of Nvidia H100 GPU resources, maximizing their utilization.
- Adaptive to Diverse AI/ML Jobs: Whether it’s model training, inferencing, or LLM training, Federator.ai GPU Booster tailors GPU resources to the specific demands of each workload, enhancing performance.
- Reduction in Resource Wastage: Federator.ai GPU Booster minimizes resource wastage by accurately predicting GPU requirements, ensuring that AI/ML jobs don’t consume more GPU power than necessary.
Accelerated AI/ML Job Completion
- Reduced Completion Time: With intelligent resource allocation, AI/ML jobs on large GPU servers are completed more swiftly, accelerating the overall workflow.
- Competitive Edge in LLM Training: The efficiency in GPU utilization mainly benefits LLM training workloads, which are resource-intensive, leading to faster model development.
Cost-Effective Operations
- Optimized Resource Spending: Better GPU utilization translates to cost savings, as more workloads can be processed with the same resources.
- Scalability and Flexibility: Federator.ai GPU Booster’s adaptability to various workloads and apply to different GPU servers make it a cost-effective solution for growing AI/ML demands.
Final Thoughts: The Future of AI/ML Workloads and Resource Optimization
Using Federator.ai GPU Booster to manage large GPU servers represents a significant stride in AI/ML workload management. Looking ahead, the future of AI/ML resource optimization is poised for transformative growth:
- Advancements in AI Algorithms: As AI algorithms become more sophisticated, the demand for efficient resource management tools like Federator.ai GPU Booster will escalate, especially for complex tasks like LLM training.
- Broader Industry Applications: With the versatility of Federator.ai GPU Booster and the availability of large GPU severs with advanced GPUs, it is expected to see a broader adoption across industries like healthcare, finance, and autonomous technologies.
- Focus on Eco-Efficiency: As environmental concerns become paramount, tools like Federator.ai GPU Booster that maximize resource utilization efficiently will play a crucial role in developing sustainable AI/ML practices.
The deployment of Federator.ai GPU Booster has resulted in 50% decrease in job completion duration, coupled with a more than twofold increase in average GPU utilization efficiency, showcasing its transformative impact.
References
- Supermicro, “SYS-821GE-TR4H, GPU Server – 8U, Dual Socket P+ (LGA 4189), Intel Xeon Scalable Processors, Supports up to 2TB Registered ECC DDR4 3200MHz SDRAM in 16 DIMM slots, Supports 8 NVIDIA H100 SXM GPUs.” Supermicro. [Online]. Available: https://www.supermicro.com/en/products/system/gpu/8u/sys-821ge-tnhr.
- Supermicro, “Supermicro GPU Systems – The Most Advanced Solutions for Deep Learning/AI, HPC, and Cloud Computing.” Supermicro. [Online]. Available: https://www.supermicro.com/en/products/gpu.
- NVIDIA, “NVIDIA H100 Tensor Core GPU – The Engine of the World’s AI Infrastructure.” NVIDIA. [Online].
Available: https://www.nvidia.com/en-us/data-center/h100/. - Oleg Zinovyev, “Managed Kubernetes with GPU Worker Nodes for Faster AI/ML Inference.” The New Stack. [Online]. Available: https://thenewstack.io/managed-k8s-with-gpu-worker-nodes-for-faster-ai-ml-inference/.
- ProphetStor, “Federator.ai Solution Granted Patent for Application-Aware, Resilient, and Optimized IT/Cloud Operations,” ProphetStor, 15 Feb. 2023. [Online]. Available: https://prophetstor.com/2023/02/15/federator-ai-solution-granted-patent-for-application-aware-resilient-and-optimized-it-cloud-operations/.
- W. X. Zhao, et al., “A Survey of Large Language Models,” arXiv, 2023. [Online]. Available: https://arxiv.org/pdf/2303.18223.
- Meta’s Llama2: “The next generation of Meta’s open source large language model.” Meta. [Online] Available: https://ai.meta.com/llama.
- OpenAI’s GPT model introduction: “Improving Language Understanding by Generative Pre-Training.” OpenAI. [Online]
Available: https://cdn.openai.com/research-covers/language-unsupervised/language_understanding_paper.pdf.