Cloud instances offer a flexible and scalable approach to workload management, as new instances can be easily launched to accommodate application growth. However, it is crucial for both optimal performance and cost savings to launch appropriate types and numbers of cloud instances to fit applications’ requirements.
With the help of machine learning-based analysis, Federator.ai can classify workload patterns for individual applications in no time, after receiving historical data, and offer appropriate portfolios of cloud instances to fit application demands. Federator.ai not only can ensure optimal performance for applications relevant to primary workload metric by providing enough resources, including the number of CPUs, memory, storage, and network capacity of cloud instances, but it can also prioritize the allocation of cheaper reserved instances and spot instances for certain applications in order to achieve cost-effective combinations of instances.
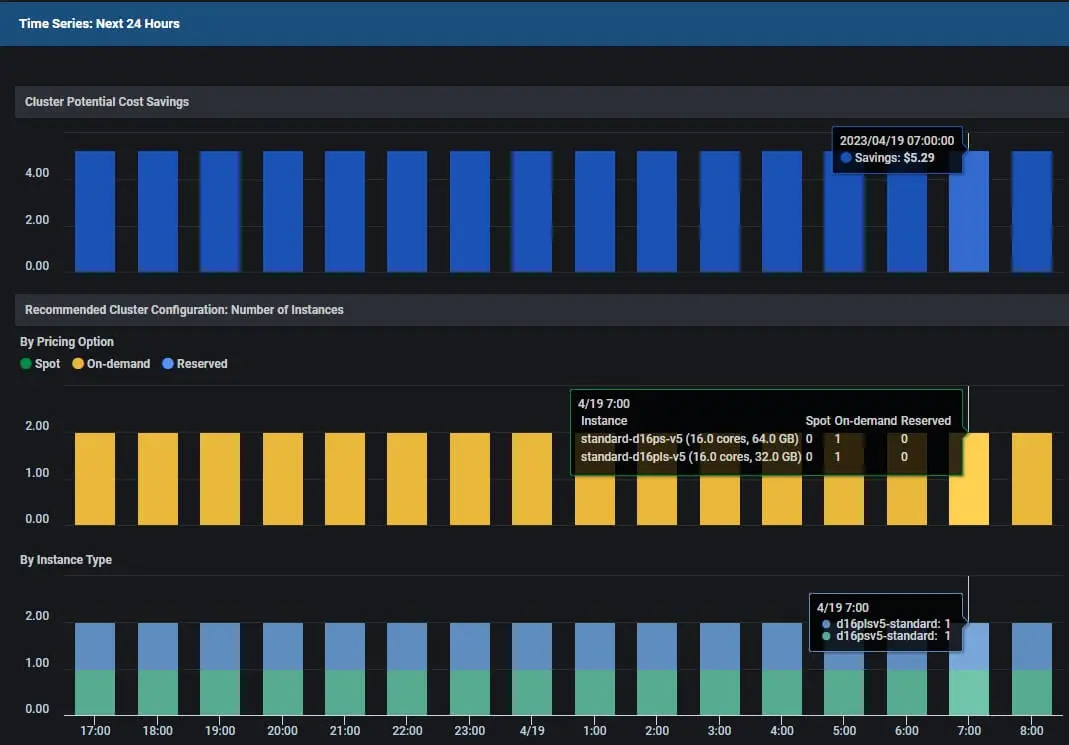
Federator.ai can achieve both the application resilience and cost savings by quickly identifying, classifying, and predicting the application workloads with its machine learning-based analysis
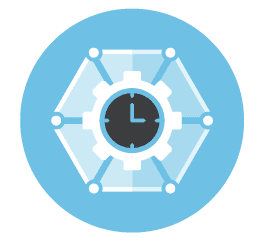
Enhanced Application Resilience
By leveraging ML-based accurate workload predictions and identifying the characteristics of each application, Federator.ai ensures optimal performance resilience by allocating the right instance types to each application at the right time.
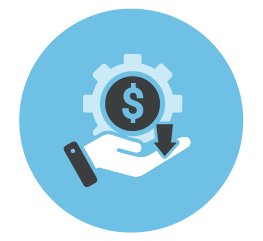
Optimized Cost Savings
Federator.ai maximizes cost savings by increasing the proportion of reserved and spot instances over on-demand instances, which reduces the cost of cloud instance procurement and provides optimal portfolio recommendations.
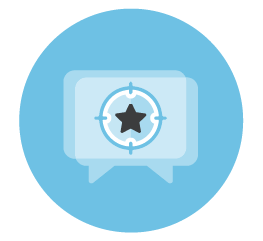
A win-win sales strategy for MSPs
Example Scenarios for MSP
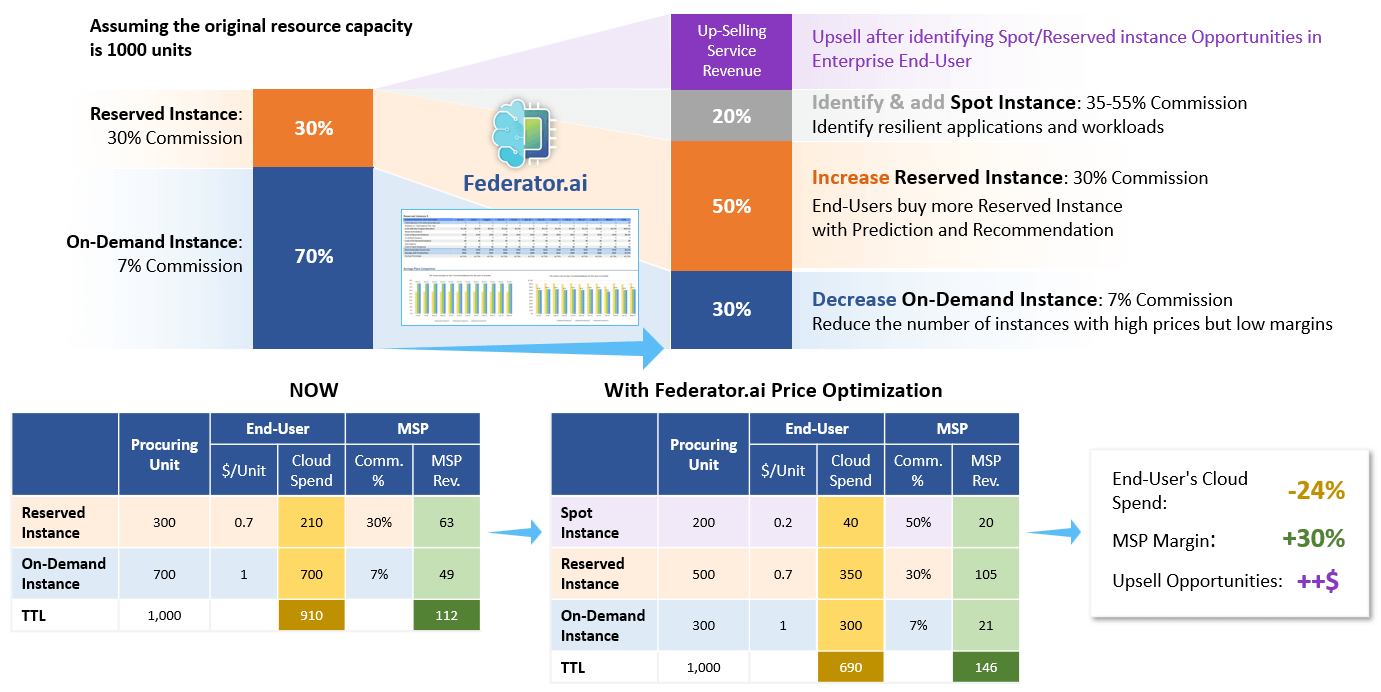
Video | Win-Win Sales Strategy for An MSP and Its End-Users
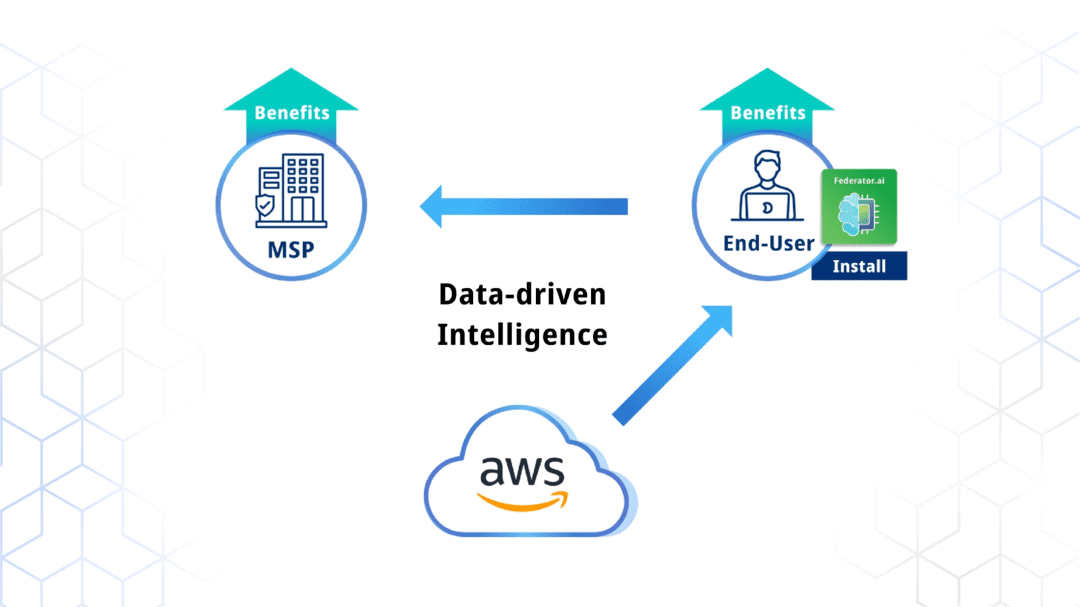
Cody Richard
CEO of Nextlink
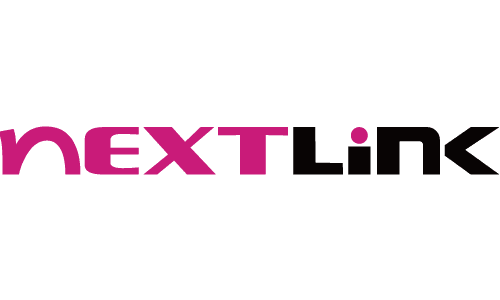