By using machine learning technologies of CrystalClear Time Series Analysis Engine, Federator.ai scales the number of containers/pods (replicas) based on predictions capturing the dynamics of application workloads to meet the resource demands by providing Just-in-Time Fitted allocation recommendations.
Based on the application-aware insight that digs into the metrics of individual applications and CPU/memory usage, Federator.ai helps users achieve much better performance (Kafka: reduce latency; NGINX: reduce average response time & HTTP response error rate ) with much fewer resources (Kafka: reduced number of Kafka consumers; Generic: CPU & memory management).
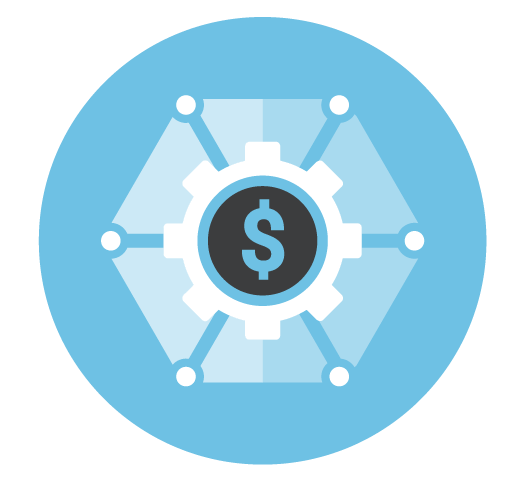
Cost-effective application deployments
Federator.ai integrates the workload metrics, workload predictions, and application KPI in deciding the right number of replicas and achieves more cost-effective application deployments.
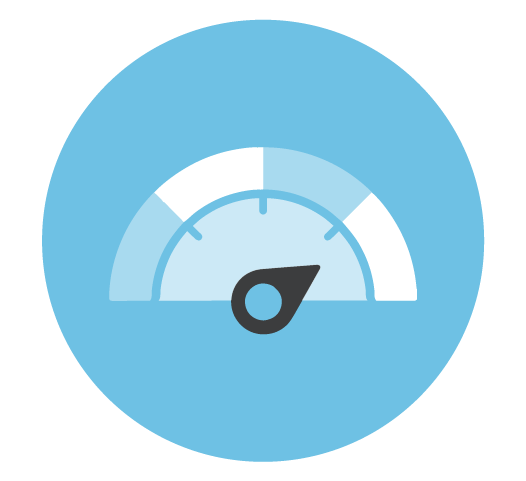
Achieving desired performance
Without guessing or experimenting on what metric threshold to set in Kubernetes native HPA, Federator.ai achieves better use of resources for desired performance automatically.
Not Just Cloud Cost Saving, Application Residence! Federator.ai is the only solution that takes care of saving the cloud cost and ensuring application resilience at the same time.
Philip Roberts
CEO of Cloudshape
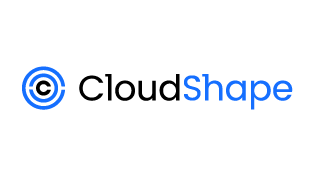