Training AI, ML, and Large Language Models (LLMs) poses significant challenges due to their resource-intensive and unpredictable demands. These workloads often lead to resource imbalances, higher costs, and scalability issues, especially in large-scale GPU clusters where utilization is hard to optimize. The dynamic nature of training complicates resource forecasting, leading to either underused infrastructure or performance bottlenecks.
To address these challenges, Federator.ai GPU Booster utilizes patented AI-powered algorithms to capture the nuances of training workload patterns and optimize GPU resource allocation across clusters. It intelligently balances resources based on real-time demand and performs seamless pod migrations within Kubernetes environments to ensure minimal downtime and optimal efficiency. By supporting various Kubernetes platforms, Federator.ai GPU Booster provides a robust, application-aware solution that streamlines AI training operations, reduces costs, and maximizes GPU utilization across diverse infrastructures.
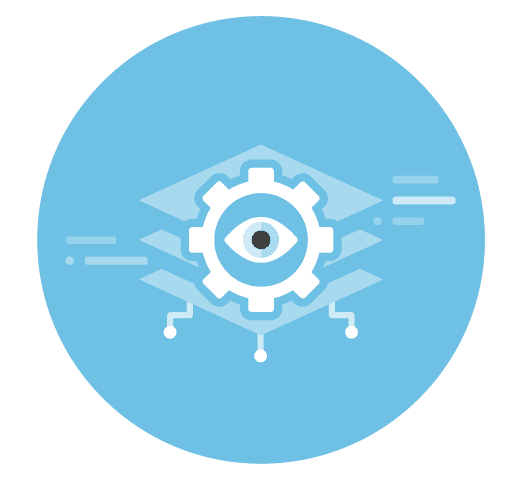
Full-Stack Visibility and Optimization
Tap into metadata and operational metrics from GPU hardware, the Kubernetes platforms, operators, AI/ML libraries, and frameworks to comprehensively view resource allocation and consumption, enabling informed resource optimization.
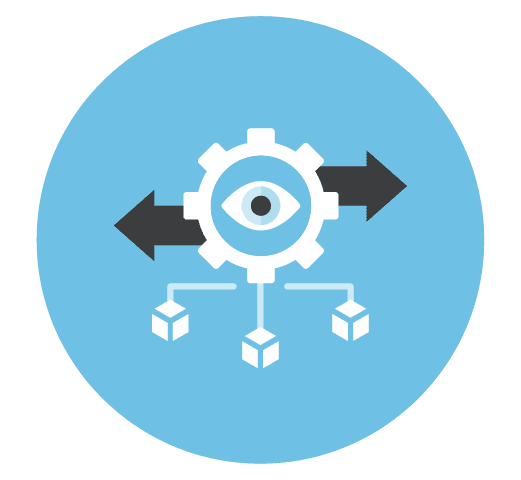
Hyper-Efficient Training Throughput
Agilely dispatch resources to support parallel MultiTenant AI/ML training and seamlessly migrate containerized applications in Kubernetes systems, avoiding performance disruption while significantly reducing training time and maximizing GPU utilization.
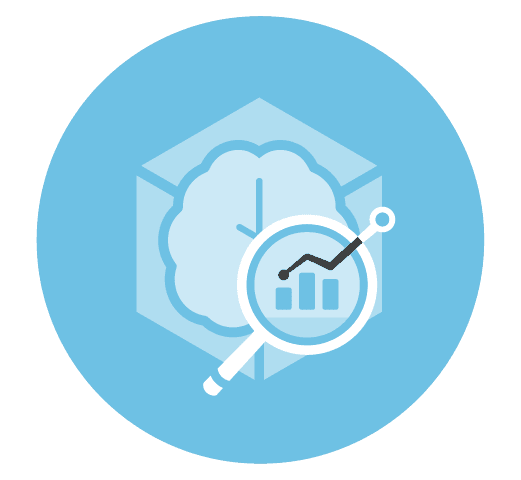
AI/ML Workload Pattern-Aware Insights
Leverage patented Spatial and Temporal GPU Optimization to multidimensionally predict resource needs for parallel AI/ML jobs, and use Cascade Causal Analysis to identify resource correlations for optimal, application-aware allocation.
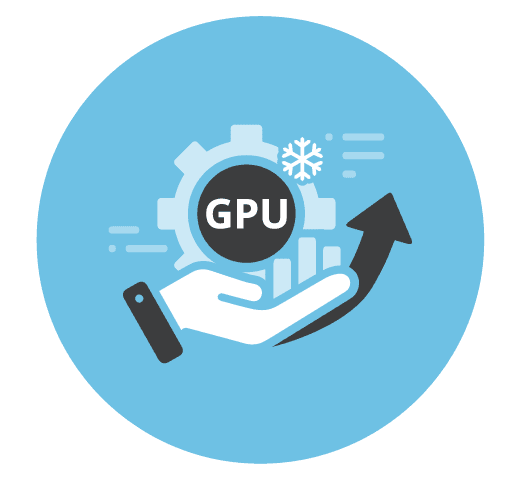
ESG Compliance for Sustainability
Capture resource demands from bursty AI training traffic to efficiently allocate GPU resources for AI/ML workloads and intelligently manage cooling for GPU servers, ensuring high GPU utilization during AI training and reduced power consumption.