Using the machine learning capabilities of CrystalClear Time Series Analysis Engine, Federator.ai predicts application workload dynamics to scale containers/pods (replicas) and provides Just-in-Time Fitted allocation recommendations.
By leveraging application-aware insights into individual applications metrics and CPU/memory usage, Federator.ai improves performance (e.g., reducing latency in Kafka, lowering average response time & HTTP error rate in NGINX) while minimizing resource usage (e.g., reducing Kafka consumers, optimizing CPU & memory management).
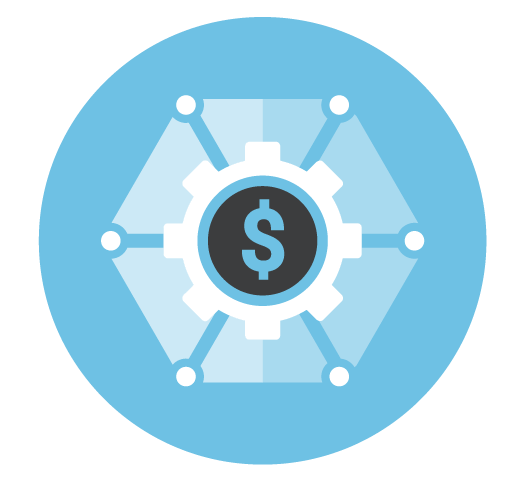
Cost-effective application deployments
Integrate workload metrics, predictions, and application KPIs to determine the optimal number of replicas, enabling more cost-effective application deployments.
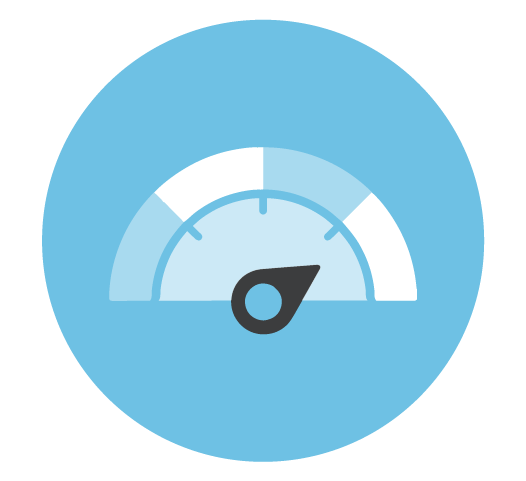
Achieving desired performance
Eliminate the need for manual threshold setting in Kubernetes native HPA by automatically optimizing resource usage to meet desired performance targets.
Philip Roberts
CEO of Cloudshape
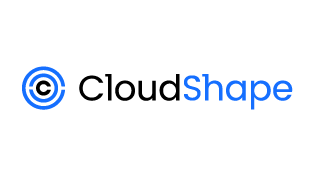